Let’s say that Mark Benioff, Mark Zuckerberg and Sayta Nadella, to name a few, are correct that artificial intelligence is poised to shake up business technology and massively automate knowledge-based tasks that we pay humans to do today. What are the implications for customer service leaders?
Customer service probably is the business function impacted the most by AI, yet specific conversations are few and far between. So, over the past few months, we’ve taken a deep dive on the question, hosting panel discussions, participating in conferences and elevating our own machine learning algorithms to new levels (stay tuned). And while there are no pat answers, some themes are emerging from the conversations that are worth sharing even if it’s for no other reason than to provoke further conversation.
[What do people mean by artificial intelligence, conversational interfaces and the other AI terms? See Definitions below.]
Here are some of the key themes and their implications for customer service leaders:
1. Outsourcing will be the first to go
Which corporate knowledge workers will see their jobs automated by machines first? “Let’s look at anything we outsource; if you outsource it, you can probably automate it,” said noted author and academic Tom Davenport1 at the recent O’Reilly AI conference in New York. “Call centers are likely to be at least a partial fatality here.”
That’s because outsourced workers typically resolve the repetitive kinds of questions that third-party agents — people who aren’t typically hands-on users of the product — can handle using scripts and knowledge databases. These are perfect questions for intelligent automation.
What’s the upshot? With a typical business process outsourcing (BPO) contract running 4.1 years2 and the pace of AI automation ramping up quickly, it makes sense for customer services leaders to question whether to sign new, long-term BPO contracts until they have more visibility into the pace of AI adoption. To reduce reliance on outsourcing, a combination of insourcing and more flexible models provided by on-demand platforms could be a more flexible alternative.
2. Smart humans will augment smart machines, indefinitely
Google is predicting that its new AI-driven Assistant will become “a predictive, all-knowing, super helpful and conversational assistant”, but Davenport and a host of PhD speakers at the O’Reilly conference agreed that while knowledge-based tasks increasingly will be automated, most organizations will need a human alternative to automation for the foreseeable future. There are many reasons for this conviction, and here are three that came up often:
1) the sheer volume of edge cases make total automation unlikely, so people will have to step in to handle specialized areas that are too narrow to automate
2) someone has to build, train and monitor the machines and use human intuition to flag the need for changes in the rules or models
3) some tasks require real people because they require social skills, creativity, context and intuition that machines may or may not achieve some day.
The keywords here are “smart humans” and “augmenting”. When machines automate the most repetitive knowledge tasks, the augmenting human layer has to be smart in different ways than the machines. This has big implications for retraining existing customer service staff so they add value on top of the automation and for tapping new sources of high-quality knowledge and talent in more economical ways.
3. Incremental approaches make sense
While customer service AI chatbots that can converse in a human-like way make for irresistible stories, panelists at our recent discussion agreed that they are not ready for prime time. Meanwhile, there are several ways to start blending human and machine intelligence to make customer service better today3 and to lay the groundwork for the future.
One example is triaging uncategorized or miscategorized questions using products that take advantage of AI and natural language processing. Another is using machine learning to suggest answers to agents, who use their own judgement about whether it’s a fit for the question. In these two examples, the AI is in the background and the interaction is human-to-human.
A third use case is a blended approach at the interface layer, where both humans and AI contribute to a chat or conversational interface4. In early applications, this typically works using a combination of structured phrase matching — a less sophisticated form of natural language process that works with large data sets and predictable keyword categories — along with on-demand human experts to fill the gaps when queries don’t match the predetermined keywords.
A great example of a text-based conversational interface with bot and human messengers, both supported by AI software, is the Lola travel app which I recently used to book business travel.
To use Lola, I simply say what my plans are, in this case, heading to a meeting in Miami followed by a flight to Newark. A bot greets me and alerts a team of on-demand travel agents. Over the next day, a team of agents asynchronously takes care of everything –researching, booking, confirming flights, hotels, restaurants — with AI helping in the background. Periodically, agents message me for clarity on details like whether I want a king or queen-size bed, and all the messages stay in one thread. Compared to doing it myself using travel sites, the experience is effortless and it saves me a lot of time. And the AI will increasingly learn my preferences and take over more of the initial steps, like suggesting flights.
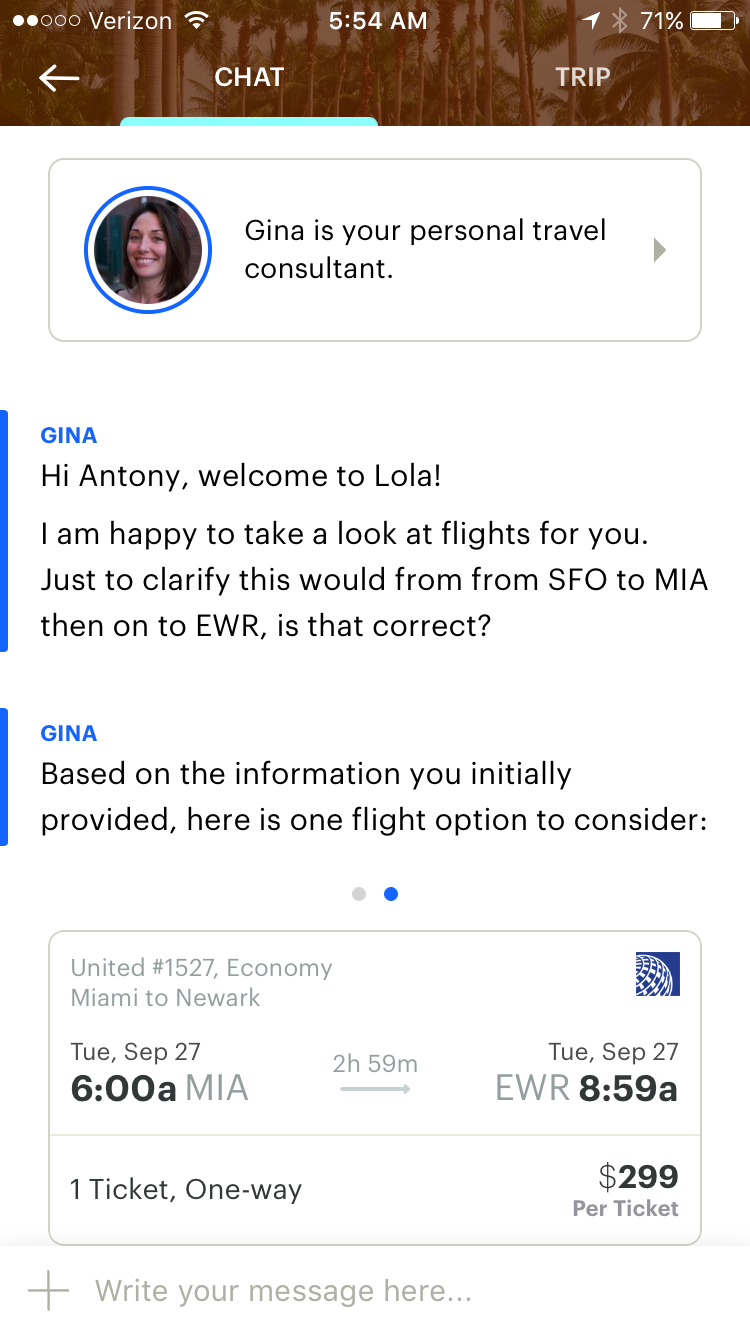
We’re excited about the human + AI conversational interface approach this example illustrates. It’s a great model for future-proofing now for tomorrow’s AI-infused world of customer service.
Definitions
What do we mean by artificial intelligence, machine learning and the other terms flying around? Here are some good sources:
For artificial intelligence definitions and influencers, check out Slate’s handy cheat sheet compiled by Jason Brogan.
For interface definitions of chatbots, messenger bots, virtual assistants, conversational UI, and natural language interfaces, check out Samrat Vasisht’s thoughtful interface category piece on Venturebeat.
1.Tom Davenport’s impressive full bio is here: www.tomdavenport.com.
2. KPMG Global IT-BPO Outsourcing Deals Analysis, Feb. 2016
3. AI can also make customer service worse, with bad interactive voice response (IVR) being an leading example.
4.
Conversational interfaces can be text-based, like webchat and
messaging, and voice-based like Apple Siri and Amazon Echo, or both.